Where does the AI semiconductor ecosystem go from here?
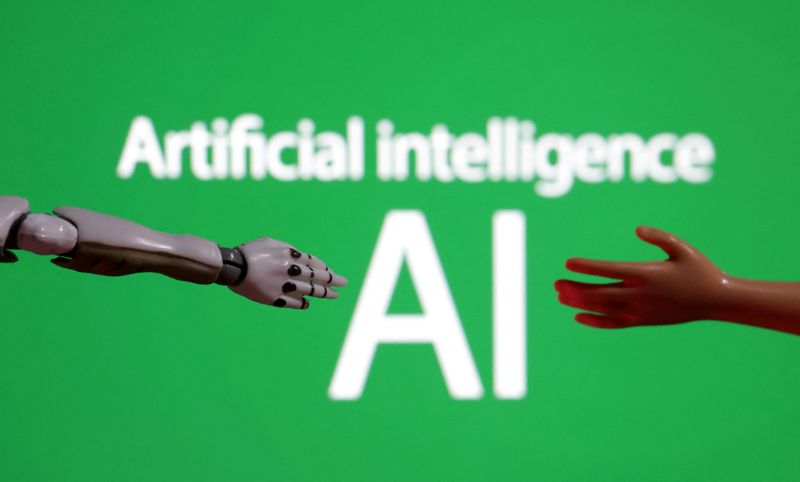
Investing.com — The trajectory of the AI semiconductor ecosystem is marked by an evolving landscape, driven by the burgeoning demand for computational power needed to fuel artificial intelligence advancements.
As per analysts at Barclays, the sector stands at a critical juncture as the global appetite for AI-powered solutions, particularly large language models, continues to outpace existing chip supply and performance.
The sell-off of AI chip names, like NVIDIA (NASDAQ:NVDA), following earnings reports has raised concerns about whether the market has reached its peak.
However, Barclays contends that the industry’s future is still rife with growth, propelled by the ever-increasing computational needs of AI models.
Barclays flags that the AI semiconductor ecosystem is in the early stages of ramping up, and this period is characterized by significant supply constraints.
The projections indicate that the compute resources required to train the next generation of LLMs, some as large as 50 trillion parameters, are monumental.
The brokerage’s estimates suggest that by 2027, nearly 20 million chips will be needed solely for training these models. This figure underscores the stark reality that AI compute demand is growing at a much faster pace than current chip technology can keep up with, even as the performance of AI accelerators improves.
The gap between AI compute demand and chip supply becomes even more evident when looking at the training requirements for models such as GPT-5, which is expected to require a 46x increase in compute power compared to GPT-4.
Yet, during this same period, the performance improvement of leading-edge chips, like NVIDIA’s next-gen Blackwell, is expected to be only sevenfold.
Compounding this issue is the limited chip production capacity, with Taiwan Semiconductor Manufacturing Company (NYSE:TSM), for instance, constrained to a production output of around 11.5 million Blackwell chips by 2025.
Adding to the complexity is the forecasted demand for inference chips. Inference, the stage where AI models generate outputs after being trained, is set to consume a large portion of the AI compute ecosystem.
Barclays notes that inference could represent up to about 40% of the market for AI chips, as evidenced by NVIDIA’s claims that a major portion of its chips are being utilized for this purpose. The overall demand for chips in both training and inference could exceed 30 million units by 2027.
As the industry grapples with these challenges, Barclays suggests a dual-track approach to the AI accelerator market, where both merchant and custom silicon solutions can thrive.
On one hand, companies like NVIDIA and AMD (NASDAQ:AMD) are well-positioned to supply chips for large-scale, frontier AI model training and inference. On the other hand, hyperscalers—companies that operate massive data centers—are likely to continue developing custom silicon for more specialized AI workloads.
This bifurcated approach will allow for flexibility in the marketplace and support various use cases outside of the large LLM realm.
Inference is expected to play an increasingly critical role, not only as a driver of demand but also as a potential revenue generator.
New methods of inference optimization, such as reinforcement learning applied in OpenAI’s latest “o1” model, signal the potential for breakthroughs in AI performance.
With better resource allocation and cost-effective inference strategies, the return on investment for AI models could improve significantly, providing incentives for continued investment in both training and inference infrastructure.